There’s an article in WSJ about the imminent ‘AI Boom’ and how a couple of companies, the ones that have already been both enabling and controlling our existence, to a large extent, are bound to become even more powerful.
It’s interesting to ‘see’ the interplay if you’ve been working in computing technology for the past decade or so. Christopher Mims, the author of the WSJ article, posits that the computing requirements of large language models, latent text-to-image diffusion models and so on, are so high that only the very few companies with massive data centers in their possession could afford/succeed in making them trustworthy. It’s not merely about computing power to train (while it is true that training such models requires many powerful computers working in tandem, the work of both academic and not-for-profit groups working on similar models in the open, with minimal funding, has shown that it’s feasible, even if you have but a fraction of the resources and wealth Google or Microsoft possess. The work of eleuther.ai and CompVis group at LMU Munich and their spin-off, stability.ai that released Stable Diffusion in 2022 — and continues to evolve it — is a testament of how much we could have without depending on the technology industry behemoths). It’s certainly not computing power to infer (the image adorning this post was generated by my personal workstation, a relatively high-end desktop computer with a few thousand dollars worth of hardware; nothing fancy or far beyond the reaches of a relatively well-off individual, let alone a company of any size).
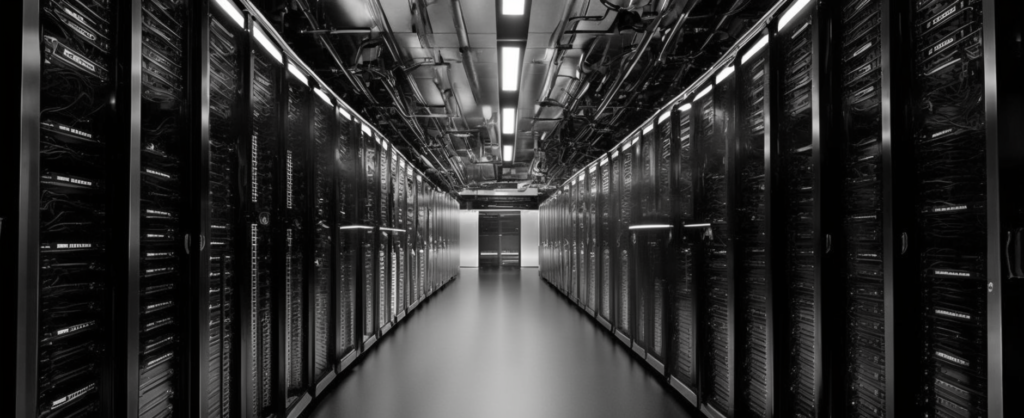
It’s the power to make said AI-powered outputs trustworthy output. Mims quotes Tinglong Dai, a professor of operations management at Johns Hopkins, who — in addition to training and serving the models at scale — considers testing and tuning these models, in order to make sure they’re not spouting an inordinate amount of nonsense or biased and offensive speech, as a reason for which only megacorps with thousands of dedicated scientists and engineers tasked with validating outputs, tuning models, attaching constraints etc. will be well positioned to render the benefits of the AI boom trustworthy.
I’m not convinced by Mims, or his interviewers’, theses. It is absolutely true that the whole notion of trust will need to be updated, radically so, in the coming years, as more and more content will be generated by computing systems, vs humans. In such a world, attribution becomes a tenuous concept, reputation mechanisms collapse. Interestingly, while both Dai and Mims mention Wikipedia, a great example of how a decentralized (in terms of authorship, not hosting etc.) encyclopædia manages to overcome both quality and bias issues through continuous moderation, editing and collaboration, they somehow fail to see how such an approach could be a great alternative (and a great foundation) to trusting large corporations — and in this case corporations with a very substantial history of failure in providing society with valid, verifiable, and ultimately trustworthy data/content.
Decentralization is never easy, that’s how a largely decentralized Web 2.0 in the mid-2000s became a vastly centralized Web 2.0 a few short years later (at all levels, from hosting/cloud computing being dominated by initially one, then a few vendors, to applications being dominated by a handful of platforms owned by even fewer corporations). Yet the computational complexity of training, using and vetting AI is nothing but a technical detail, no different from the plethora of other details we have so keenly ignored while enduring the barrage of centralization in our lives in the past fifteen years or so. Computing power increases, sometimes non-linearly and what seems like a daunting task for personal computing systems today might seem trivial a few years from now. We have been willfully ceding control and privacy away to large corporations for years, in spite of there being hardly a technical reason to support it (from smart home, to smart ‘assistants’, to cloud-hosted business and personal applications and storage) — on the contrary, you could argue that cloud-based dictation is harder on mobile networks than it is to achieve locally, with modern ‘accelerated neural network’ CPUs being part of every phone, tablet and PC manufactured in the past few years!
It will be more a matter of willingness, on the part of us, the end-users, alignment, on the part of all involved stakeholders, and the creation and ubiquity of open, freely available, self-hosted and self-managed, easy to use solutions, offering similar if not superior performance to those on offer by “Big Tech”, than it will be a technical challenge.